As a Customer Success/Delivery Manager I am not too technical and always try to get a better understanding of what AIOps actually is and means for organizations. I started with this wikipedia article , but to be honest it is still a bit vague to me. In my role, I have the benefit to be in direct touch with our customers all the time and learn what AIOps actually means for them. Especially the value it delivers. To put it simply, ‘AIOps is the application of machine learning and data science to IT operations problems’. I would like to use this blog article to share the top three trends and AIOps journeys directly from the field with you.
1. Instant visibility
It all starts with data - what a cliché, right?! One of the biggest challenges customers face today, is to add visibility on top of their data. All the data is there, but it's scattered across different sources, tools or is centralized in a single source, for example a data lake in Splunk. The challenge is the same: IT teams not only need access to this data, but they also need visibility into the relationships and dependencies that exists across this data to be able to draw out meaningful conclusions. Understanding the context of your data and knowing what your IT landscape looks like, is crucial to go forward.
The data that StackState is typically looking for can be categorized into two areas: 1) topology and 2) telemetry data (such as metrics, logs and events). Because StackState's AIOps platform is ‘data-agnostic’, it is able to integrate with 100+ different tools and data sources. StackState uses the data it collects to learn about dependencies, allowing it to build a topology of a user’s IT environment. All in an automated way. As one of our MSP customers stated, ‘I simply want to put the plug into my customers environment and want to have instant visibility’ and this is exactly where the AIOps journey starts at our customers.
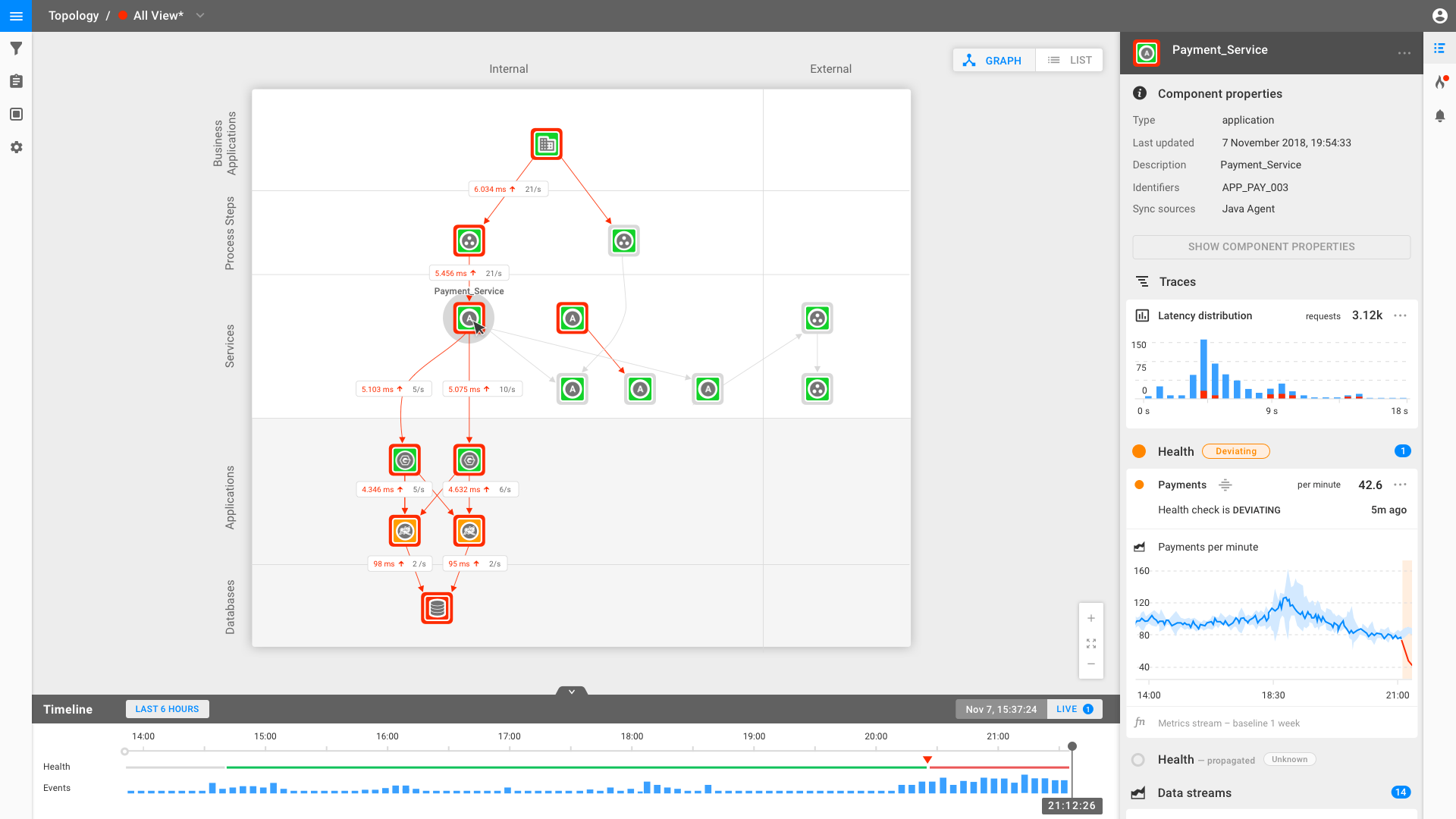
2. Full IT stack monitoring in relation to business processes
End-to-end monitoring is a term which is often used and also relates to StackState. The first thing I always ask myself is what the definition of end-to-end exactly is? A trend I definitely see and also seems to be common sense in our customer implementations, is not to only use IT related data, but also data related to business processes and operations. The latter usually contains business KPI’s and becomes very valuable if the relation and dependencies to data from the IT environment are visualized. An example of business related data is the integration with Google Analytics. By combining these two different types of data, you are able to predict the impact and risk on business processes, if a failure or outage occurs in the underlying IT infrastructure. Automatically relating IT issues to business performance is one of the key use cases I see at our customers.
3. Become predictive
Consolidating different data sources in one platform, visualizing dependencies between IT and business KPIs and configuring the 'traffic lights' (which we call checks in StackState) enables you to do fast root cause analysis and reduce the mean-time-to-repair to a minimum. A quick ROI is established by using the power and flexibility of StackState's AIOps platform.
"So, what is the Artificial Intelligence part of AIOps?" I hear you thinking.
By talking to and learning from my customers, their common goal is to become predictive. They want to receive an alert before a problem occurs, so that it can be fixed before impacting the business and before customers start complaining. And StackState is well-positioned to achieve this goal.
Artificial Intelligence needs context. It's hard to draw insights while being restricted to only one source of information without the necessary context. As I explained before, StackState is able to provide you with instant visibility and full stack monitoring across the entire landscape. It enables IT operators to understand the data in a broader context. Currently StackState's algorithms enables the following three capabilities:
Automated root cause and impact analysis: get instant insight into the cause and (business) impact of IT issues.
Problem clustering: automatically identify issues that are likely to related in cause and cluster them together, so you aren’t deluged with cascades of individual alerts when problems occur.
Anomaly detection: static checks doesn't work for today's dynamic environments. Anomaly detection does the work for you and automatically sets up baselines. If there's any anomalous behavior, you receive an alert.
And this isn't everything yet. Our vision goes one step further: the automated pre-mortem analysis.
If you ask me, this is exactly where the simple explanation about AIOps: “…the application of machine learning and data science to IT operations problems” starts to make sense. This is our customer's journey towards AIOps together with StackState.
If you want to learn more about how you and your organization can get started with AIOps, get in touch with us or request a 20-minute demo of our full stack observability platform.