DOWNLOAD THIS BLOG AS PDF - Handy when you don't have the time now or want to access it anytime you want!
Data in separate silo's blinds decision making
Gartner introduces the problem by pointing out that most I&O leaders use Artificial Intelligence for IT Operations (AIOps) for event correlation and analysis on the one hand. On the other hand, the CMDB is used for change and configuration management. As a consequence, data about running the business (Mode 1) and data for growing the business (Mode 2) is stored separately. This disables I&O leaders to understand change without the dimension of performance, and performance without the overlay of service and change, Gartner notes. These changes could then lead to conflict and harming critical IT systems and business services, impacting organization’s ability to deliver on digital transformation.
However, by starting with AIOps, adding your CMDB and using both working in concert, Gartner describes a way for I&O leaders to reduce the adverse impacts of planned changes while improving customer experience, service health and business results. Following these three steps.
Step 1: Use AIOps to do a pre-mortem analysis
AIOps can help you assess the risk of any changes without using a test environment. By applying the pattern-matching algorithms of AIOps technologies to historical change data, an insight can be given into the consequences that type of change had in the past. Gartner advises to use this analysis to create a risk score (high, medium and low) to quantify acceptable risk and to serve as the basis for the decision to deploy changes. At StackState we like to call this a ‘pre-mortem analysis’ as opposed to the well-known post-mortem analysis.
“AIOps can be used to look across many dimensions and rationalize all of that data into actionable insight”, Gartner writes. This is impossible to do for humans. Gartner advises to use AIOps to detect the patterns that relate incidents with changes as reported by those tools.
Gartner further presents the following three action items:
Provide AIOps tools with the data necessary to detect patterns and relationships by collaborating with DevOps teams to tag, label and send updates from ARO and CCA tools. Include events from builds and deployments as part of the automated pipelines, along with IT monitoring data.
Classify the highest-risk changes (those with the greatest adverse impact) by using AIOps to identify those that repeatedly result in instability for the top two to four business-impacting applications.
Communicate the identified risk of prospective changes by comparing them to the change categories and applications that AIOps has identified as risky to ensure a more stringent evaluation.
Step 2: Use CMDB to reduce the unexpected consequences of changes
To perform the pre-mortem analysis described above, it’s important to have sufficient historical data. Therefore, Gartner advises that I&O leaders keep their historical data in a CMDB. Of course, you can also keep historical data in other repositories, like a data lake. As long as you are able to access all this data and have a platform to combine these siloed data fragments. In turn, AIOps can learn from this historical data and avoid unexpected consequences of IT change management.
But how can a CMDB help if there is no historical data? What if a change doesn’t have a precedent? How can unexpected side effects be avoided then? Gartner advises I&O leaders to continue to use the dependency mapping and configuration discovery insight that are the province of CMDB tools. CMDB tools can enable a forward-looking view of IT service performance that can facilitate planning for change and show deviations from expected results.
Step 3: Let AIOps and CMDB work in concert
Successful change management requires collaboration between DevOps, monitoring and ITSM teams Gartner states. This is not only a technical, API-driven collaboration but also a social, cooperative one. The cooperation starts with an agreement between the teams regarding agreed-upon tagging of changes: how will they be sent and in what format?
If this social, cooperative part is agreed upon within a team, it’s time to tackle the technical part. Gartner advises that the DevOps team makes sure that the Application Release Orchestration (ARO) tools send pending changes to an AIOps tool in the form of a tag-based, user-defined change event. The ITSM team must do the same with the changes it proposes as well as the topology for where the changes will occur, Gartner writes. To better understand potential impact for change it’s good to create a notion of locality by correlating topology from the CMDB with events from ARO. Gartner further advises to select AIOps tools that offer built-in capability to use topology from multiple sources, such as a monitoring tool and the CMDB, and can reconcile them for greater predictive accuracy.
That's where StackState comes in
StackState is one of those select tools that is able to offer built-in capability to use topology from multiple sources, like the CMDB and a monitoring tool. The platform applies machine learning algorithms to determine which patterns have greater relevance due to their locality. StackState can, as Gartner describes: "provide the probability that the impact of a change on one node can be propagated to another. This can help predict the likelihood of a proposed change creating an undesirable impact".
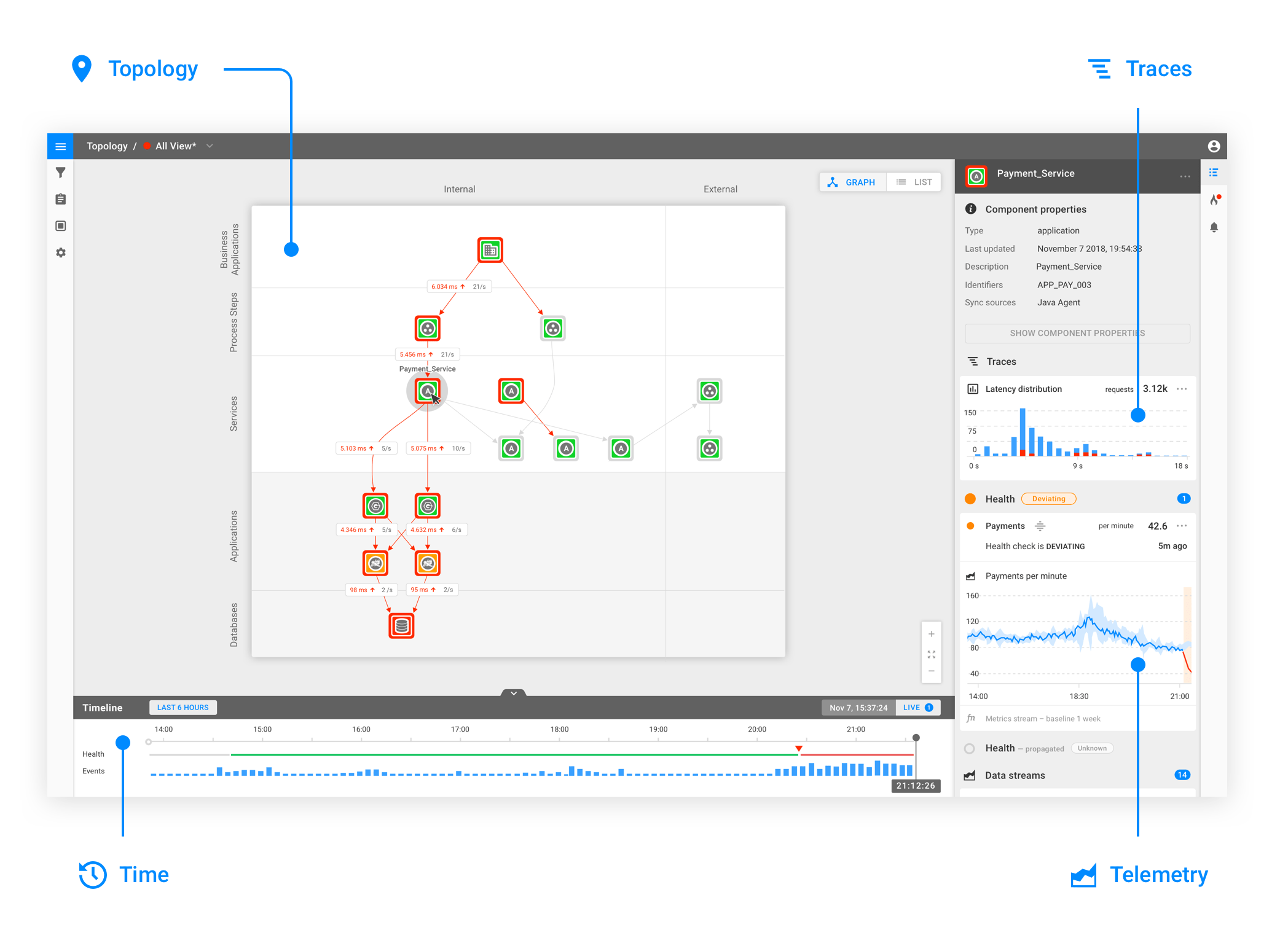
StackState is not only able to combine and correlate data from any data source, but can also keep track of all changes in the landscape. Whether it's a deployment, an infrastructure change or any other change, nothing is overlooked. You can experience StackState by booking a guided tour .
Gartner closes their report by advising the following three action items:
Use AIOps pattern analysis to shorten the time required to make an effective change by creating a continuous feedback loop between DevOps and monitoring
Update current service model definitions by using the combined data of AIOps and the CMDB to create updated service model definitions based on both topology and changes.
Measure the time to detect a change impacting a service and the accuracy in decision making regarding changes, and track improvements regularly.
About StackState
StackState is the leading monitoring and AIOps platform for hybrid IT. The platform combines and analyzes metrics, logs, events, and data beyond typical monitoring data, like Google Analytics, CMDBs, CI/CD tools, service registries, automation, and incident management tools. The 4T Data Model® is the core of StackState's monitoring and AIOps platform and the main driver for all real-time monitoring, automation, and predictive capabilities. It combines big data, artificial intelligence, and topology visualization to instantly pinpoint the root cause of (predicted) incidents and improve business alignment. The platform helps organizations make better decisions faster and avoid high severity outages while utilizing their current IT investments. StackState's growing customer list encompasses a range of industries — from finance, telecom, to managed service providers — and includes global enterprises like IBM Global Services, Vodafone, KPN Telecom, and ABN AMRO Bank as well as local innovators, such as NS International and Schuberg Philis.