Automated Root Cause Analysis & Anomaly Detection
Automated Root Cause Analysis helps IT operators reduce the mean time to repair. It allows you to see the root cause of a problem immediately and to focus on solving it. But in order for it to work properly, it needs some kind of Artificial Intelligence (AI) behind it that can figure out what's wrong with the system. Or in other words, how does Automated Root Cause Analysis get to know what the root cause of a problem is?
Have you read our latest white paper already? Download it right here!
To find out what caused a problem, Automated Root Cause Analysis looks at where the problem occurs at the moment and then uses the IT environment's topology to trace the problem to its origins. Automated Root Cause Analysis walks through the topological graph and examines each component, figuring out if it is misbehaving.
That's where Anomaly Detection comes in. Anomaly Detection compares current behavior with what it considers reasonable, and if it sees a significant deviation, it will flag the component as a potential root cause. In short, Automated Root Cause Analysis uses Anomaly Detection to decide if a component can be a root cause of a problem. That's why a solution that has a goal to prevent outages and reduce MTTR should always have both Automated Root Cause Analysis and Anomaly Detection working in concert. Not only one or the other.
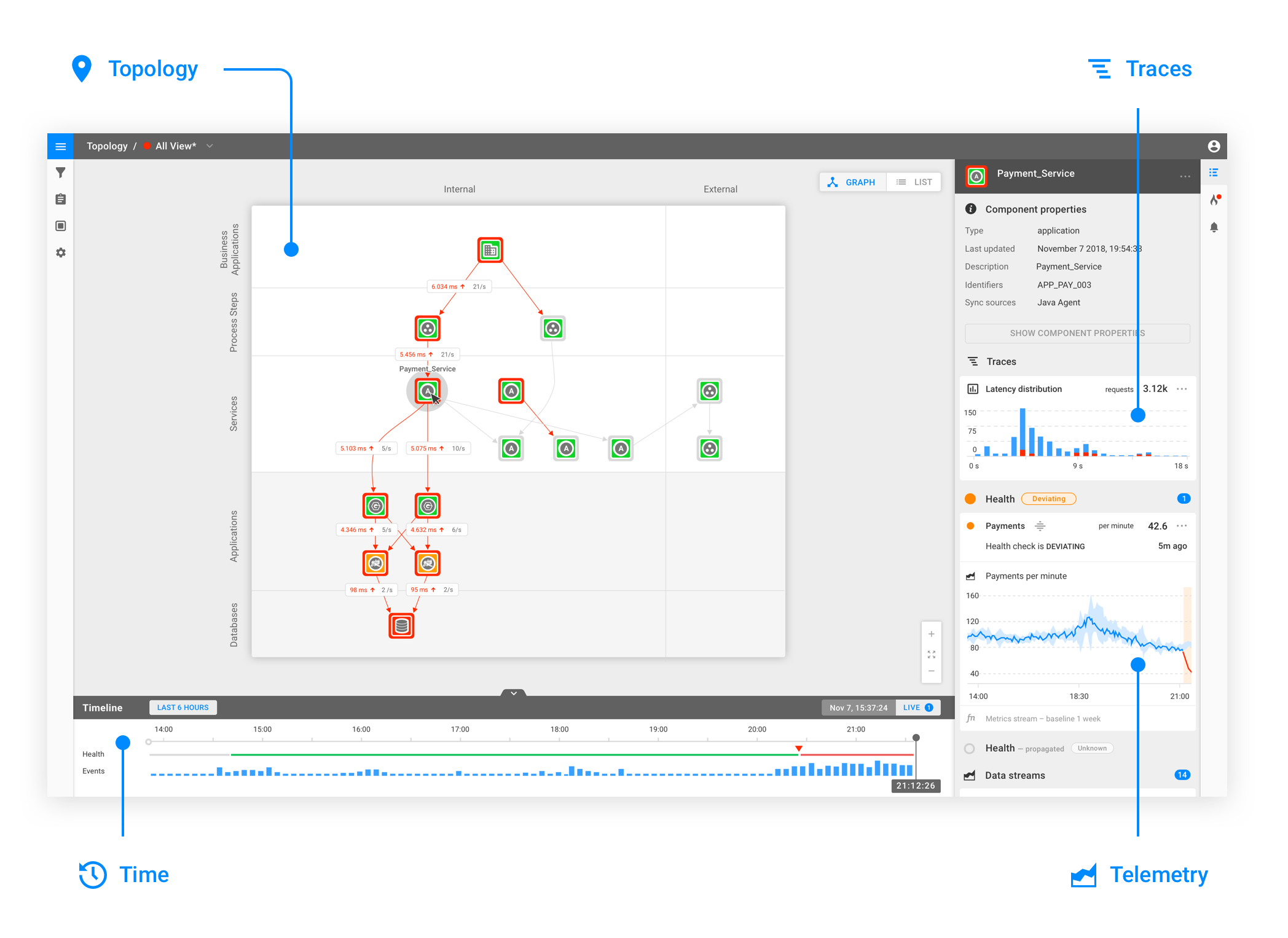
StackState's Synergy Effect
The extent to which Automated Root Cause Analysis and Anomaly Detection are efficient in solving problems can differ a lot. They have a certain level of quality. Not only that, but the relationship between them can also affect the level of quality. Because the condition of Anomaly Detection affects the quality of Automated Root Cause Analysis and the MTTR, this synergy effect is essential.
How can you get the most out of it?
At StackState, we use Automated Root Cause Analysis to tune our Anomaly Detection. StackState applies Automated Machine Learning to select Anomaly Detection algorithms to correctly point out the root cause of problems that have occurred in the past. In other words, the 'machine' is 'learning' automatically from the past. This way, it becomes better each time it identifies and solves a new issue. Using StackState's 4T data model, incidents are linked to problems and automatically improve the quality of Anomaly Detection, resulting in a better Automated Root Cause Analysis and reducing MTTR. Keep an eye on our blog to get to know more about this cool feature!
NOTE: Automated Root Cause Analysis using Anomaly Detection will be available in StackState V. 4.2
About StackState
StackState's observability platform lets your manage your dynamic IT environment by combining all performance data from existing monitoring tools into a single topology. Moreover, the time-traveling topology then lets you travel back in time to see exactly what your environment looked like before an issue popped up. The result? Peace of mind and immensely effective root cause analysis.
Curious to see how it works? Play around in our sandbox environment or sign up for a free trial to try StackState with your own data.